The 6th EMC2 - Energy Efficient Machine Learning and Cognitive Computing
description Workshop Objective
With the advent of ChatGPT and other language models, Generative AI and LLMs have captured the imagination of whole world! A new wave of intelligent computing, driven by recent advances in machine learning and cognitive algorithms coupled with processtechnology and new design methodologies, has the potential to usher unprecedented disruption in the way modern computing systemsare designed and deployed. These new and innovative approaches often provide an attractive and efficient alternative not only in terms of performance but also power, energy, and area. This disruption is easily visible across the whole spectrum of computing systems– ranging from low end mobile devices to large scale data centers. Applications that benefit from efficient machine learning include computer vision and image processing, augmented/mixed reality, language understanding, speech and gesture recognition, malware detection, autonomous driving, and many more. Naturally, these applications have diverse requirements for performance, energy, reliability, accuracy, and security that demand a holistic approach to designing the hardware, software, and intelligence algorithms to achieve the best outcome.
chat Call for Papers
The goal of this Workshop is to provide a forum for researchers and industry experts who are exploring novel ideas, tools and techniques to improve the energy efficiency of MLLMs as it is practised today and would evolve in the next decade. We envision that only through close collaboration between industry and the academia we will be able to address the difficult challenges and opportunities of reducing the carbon footprint of AI and its uses. We have tailored our program to best serve the participants in a fully digital setting. Our forum facilitates active exchange of ideas through:
- Keynotes, invited talks and discussion panels by leading researchers from industry and academia
- Peer-reviewed papers on latest solutions including works-in-progress to seek directed feedback from experts
- Independent publication of proceedings through IEEE CPS
We invite full-length papers describing original, cutting-edge, and even work-in-progress research projects about efficient machine learning. Suggested topics for papers include, but are not limited to the ones listed on this page. The proceedings from previous instances have been published through the prestigious IEEE Conference Publishing Services (CPS) and are available to the community via IEEE Xplore. In each instance, IEEE conducted independent assessment of the papers for quality.
format_list_bulleted Topics for the Workshop
- Neural network architectures for resource constrained applications
- Efficient hardware designs to implement neural networks including sparsity, locality, and systolic designs
- Power and performance efficient memory architectures suited for neural networks
- Network reduction techniques – approximation, quantization, reduced precision, pruning, distillation, and reconfiguration
- Exploring interplay of precision, performance, power, and energy through benchmarks, workloads, and characterization
- Simulation and emulation techniques, frameworks, tools, and platforms for machine learning
- Optimizations to improve performance of training techniques including on-device and large-scale learning
- Load balancing and efficient task distribution, communication and computation overlapping for optimal performance
- Verification, validation, determinism, robustness, bias, safety, and privacy challenges in AI systems
08:40 - 09:40
Keynote
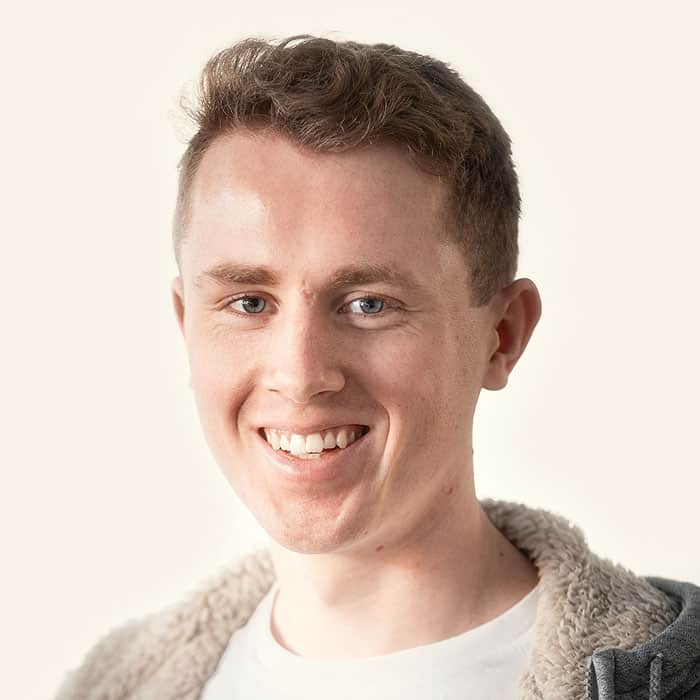
Achieving Low-latency Speech Synthesis at Scale
Sam Davis, Myrtle.ai link
Real-time text-to-speech models are widely deployed in interactive voice services such as voice assistants and smart speakers. However, deploying these models at scale is challenging due to the strict latency requirements that they face: one pass through the model must complete once every 62.5 microseconds to generate 16kHz audio. This talk will introduce these challenges through the WaveNet model, a state-of-the-art speech synthesis vocoder. It then discusses how this class of models can achieve high-throughput, low-latency inference at scale through the combination of three components: model compression, more suitable programming paradigms, and more efficient compute platforms.
Sam Davis is Head of Machine Learning at Myrtle.ai where his team works to understand and develop efficient, state-of-the-art machine learning models. His interests and work focus on the intersection of Conversational AI, model compression, and hardware design for machine learning inference. He chaired the MLPerf.org working group to develop the speech recognition benchmark that was released as part of the recent v0.7 inference round.
09:40 - 10:15
Invited Talk
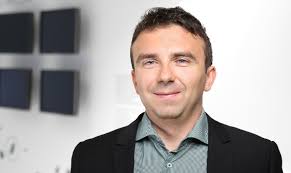
DeepCABAC: A Universal Compression Algorithm for Deep Neural Networks
Wojciech Samek, Fraunhofer Heinrich Hertz Institute link
In the past decade, deep neural networks (DNNs) have shown state-of-the-art performance on a wide range of complex machine learning tasks. Many of these results have been achieved while growing the size of DNNs, creating a demand for efficient compression and transmission of them. This talk will present DeepCABAC, a universal compression algorithm for DNNs that through its adaptive, context-based rate modeling, allows an optimal quantization and coding of neural network parameters. It compresses state-of-the-art DNNs up to 1.5% of their original size with no accuracy loss and has been selected as basic compression technology for the emerging MPEG-7 part 17 standard on DNN compression.
Wojciech Samek has founded and is heading the Machine Learning Group at Fraunhofer Heinrich Hertz Institute since 2014. He studied computer science at Humboldt University of Berlin, Heriot-Watt University and University of Edinburgh from 2004 to 2010 and received the Dr. rer. nat. degree with distinction (summa cum laude) from the Technical University of Berlin in 2014. In 2009 he was visiting researcher at NASA Ames Research Center, Mountain View, CA, and in 2012 and 2013 he had several short-term research stays at ATR International, Kyoto, Japan. He was awarded scholarships from the European Union’s Erasmus Mundus programme, the Studienstiftung des deutschen Volkes and the DFG Research Training Group GRK 1589/1. He is PI at the Berlin Institute for the Foundation of Learning and Data (BIFOLD), member of the European Lab for Learning and Intelligent Systems (ELLIS) and associated faculty at the DFG graduate school BIOQIC. Furthermore, he is an editorial board member of Digital Signal Processing, PLOS ONE and IEEE TNNLS and an elected member of the IEEE MLSP Technical Committee. He has organized special sessions, workshops and tutorials at top-tier machine learning conferences (NIPS, ICML, CVPR, ICASSP, MICCAI), has received multiple best paper awards, and has authored more than 100 journal and conference papers, predominantly in the areas deep learning, interpretable machine learning, neural network compression and federated learning.
10:15 - 10:20
Break
Break
10:20 - 10:55
Invited Talk
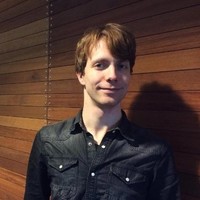
AdaRound and Bayesian Bits: New advances in Quantization
Tijmen Blankevoort, Qualcomm Inc. link
In this talk, Tijmen will introduce two new methods for quantizing your neural networks. AdaRound, which is a new rounding scheme that allows us to quantize several networks to even 4 bit weights, with a small drop in accuracy while not requiring fine-tuning. And Bayesian Bits, a fine-tuning method that allows a network to automatically choose mixed-precision quantization settings and do pruning at the same time. Both methods have successfully been applied in practice, and make neural network quantization a lot easier for engineers who want to deploy their models to energy efficient devices.
Tijmen Blankevoort is a senior staff engineer at Qualcomm, leading the model efficiency research team. From quantization to pruning and neural architecture search, his team is developing new methods to deploy neural networks in the most energy efficient way possible. Before joining Qualcomm, Tijmen has a degree in mathematics from the university of Leiden. Together with Max Welling, he started the deep learning start-up Scyfer as a spin-off from the university of Amsterdam, which was successfully acquired by Qualcomm in 2017. In his spare time, he enjoys playing the card game Magic: the Gathering, and molecular gastronomy cooking.
10:55 - 11:30
Invited Talk
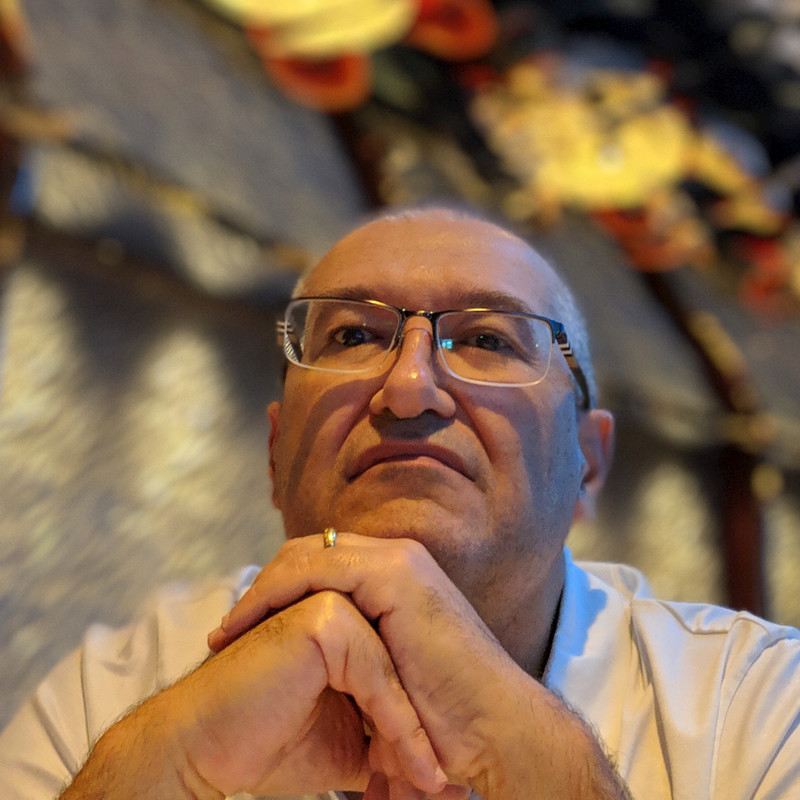
Designing Nanosecond Inference Engines for the Particle Collider
Clauidonor N. Coelho Jr (Palo Alto Networks), Thea Aarrestad (CERN), Vladimir Loncar (CERN), Maurizio Pierini (CERN), Adrian Alan Pol (CERN), Sioni Summers (CERN), Jennifer Ngadiuba, (Caltech)
While the quest for more accurate solutions is pushing deep learning research towards larger and more complex algorithms, edge devices with hard real-time constraints demand very efficient inference engines, e.g. with the reduction in model size, speed and energy consumption. In this talk, we introduce a novel method for designing heterogeneously quantized versions of deep neural network models for minimum-energy, high-accuracy, nanosecond inference and fully automated deployment on chip. Our technique combines AutoML and QKeras (which is called AutoQKeras), combining layer hyperparameter selection and quantization optimization. Users can select among several optimization strategies, such as global optimization of network hyperparameters and quantizers, or splitting the optimization problems into smaller search problems to cope with search complexity. We have applied this design technique for the event selection procedure in proton-proton collisions at the CERN Large Hadron Collider, where resources are strictly limited and latency of O(1) us is required. Nanosecond inference and a resource consumption reduced by a factor of 50 when implemented on FPGA hardware are achieved.
11:30 - 12:10
Paper Session #1
Towards Co-designing Neural Network Function Approximators with In-SRAM Computing
Shamma Nasrin, Diaa Badawi, Ahmet Enis Cetin, Wilfred Gomes and Amit Trivedi
University of Illinois at Chicago
Paper
The Architectural Implications of Distributed Reinforcement Learning on CPU-GPU Systems
Ahmet Inci, Evgeny Bolotin, Yaosheng Fu, Gal Dalal, Shie Mannor, David Nellans and Diana Marculescu
CMU, NVIDIA, UT Austin
Paper
Platform-Aware Resource Allocation for Inference on Multi-Accelerator Edge Devices
Ramakrishnan Sivakumar, Saurabh Tangri and Satyam Srivastava
Intel Corporation
Paper
AttendNets: Tiny Deep Image Recognition Neural Networks for the Edge via Visual Attention Condensers
Alexander Wong, Mahmoud Famouri and Mohammad Javad Shafiee
University of Waterloo, DarwinAI
12:10 - 12:30
Lunch Break
Lunch Break
12:30 - 13:10
Paper Session #2
Recipes for Post-training Quantization of Deep Neural Networks
Ashutosh Mishra, Christoffer Löffler and Axel Plinge
Fraunhofer IIS
Paper
FactorizeNet: Progressive Depth Factorization for Efficient CNN Architecture Exploration Under Quantization Constraints
Stone Yun and Alexander Wong
University of Waterloo
Paper
Auto Hessian Aware Channel-wise Quantization of Neural Networks
Xu Qian, Victor Li and Crews Darren
Intel Corporation
Paper
Hardware Aware Sparsity Generation for Convolutional Neural Networks
Xiaofan Xu, Zsolt Biro and Cormac Brick
Intel Corporation
13:10 - 14:10
Keynote
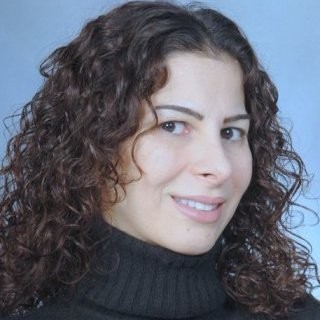
Energy Efficient Machine Learning on Encrypted Data: Hardware to the Rescue
Farinaz Koushanfar, University of California, San Diego link
Machine Learning on encrypted data is a yet-to-be-addressed challenge. Several recent key advances across different layers of the system, from cryptography and mathematics to logic synthesis and hardware are paving the way for energy-efficient realization of privacy preserving computing for certain target applications. This keynote talk highlights the crucial role of hardware and advances in computing architecture in supporting the recent progresses in the field. I outline the main technologies and mixed computing models. I particularly center my talk on the recent progress in synthesis of Garbled Circuits that provide a leap in scalable realization of energy efficient machine learning on encrypted data. I explore how hardware could pave the way for navigating the complex parameter selection and scalable future mixed protocol solutions. I conclude by briefly discussing the challenges and opportunities moving forward.
Farinaz Koushanfar is a professor and Henry Booker Faculty Scholar in the Electrical and Computer Engineering (ECE) department at University of California San Diego (UCSD), where she is also the co-founder and co-director of the UCSD Center for Machine-Integrated Computing & Security (MICS). Her research addresses several aspects of efficient computing and embedded systems, with a focus on hardware and system security, real-time/energy-efficient big data analytics under resource constraints, design automation and synthesis for emerging applications, as well as practical privacy-preserving computing. Dr. Koushanfar is a fellow of the Kavli Foundation Frontiers of the National Academy of Engineering and a fellow of IEEE. She has received a number of awards and honors including the Presidential Early Career Award for Scientists and Engineers (PECASE) from President Obama, the ACM SIGDA Outstanding New Faculty Award, Cisco IoT Security Grand Challenge Award, MIT Technology Review TR-35 2008, as well as Young Faculty/CAREER Awards from NSF, DARPA, ONR and ARO.
14:10 - 14:45
Invited Talk
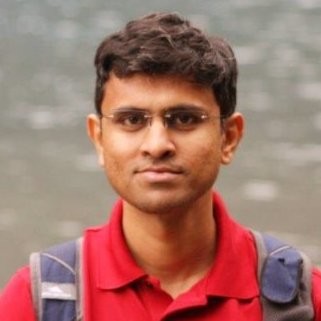
Techniques for Efficient Inference with Deep Networks
Raghu Krishnamoorthi, Facebook
Efficient inference is a problem of great practical interest for both on-device AI and server side applications. In this talk, I will talk about quantization for efficient inference and discuss practical approaches to get the best performance and accuracy when you deploy a model for inference. I will conclude the talk by touching upon sparsity which can provide further performance improvements on top of quantization.
Raghuraman Krishnamoorthi is a software engineer in the Pytorch team at Facebook, where he leads the effort to optimize deep networks for inference, with a focus on quantization. Prior to this he was part of the Tensorflow team at Google working on quantization for mobile inference as part of Tensorflow Lite. From 2001 to 2017, Raghu was at Qualcomm Research, working on several generations of wireless technologies. His work experience also includes computer vision for AR, ultra-low power, always-on vision, hardware/software co-design for inference on mobile platforms and modem development. He is an inventor in more than 90 issued and filed patents. Raghu has a Masters degree in EE from University of Illinois, Urbana Champaign and a Bachelors degree from the Indian Institute of Technology, Madras.
14:45 - 15:20
Invited Talk
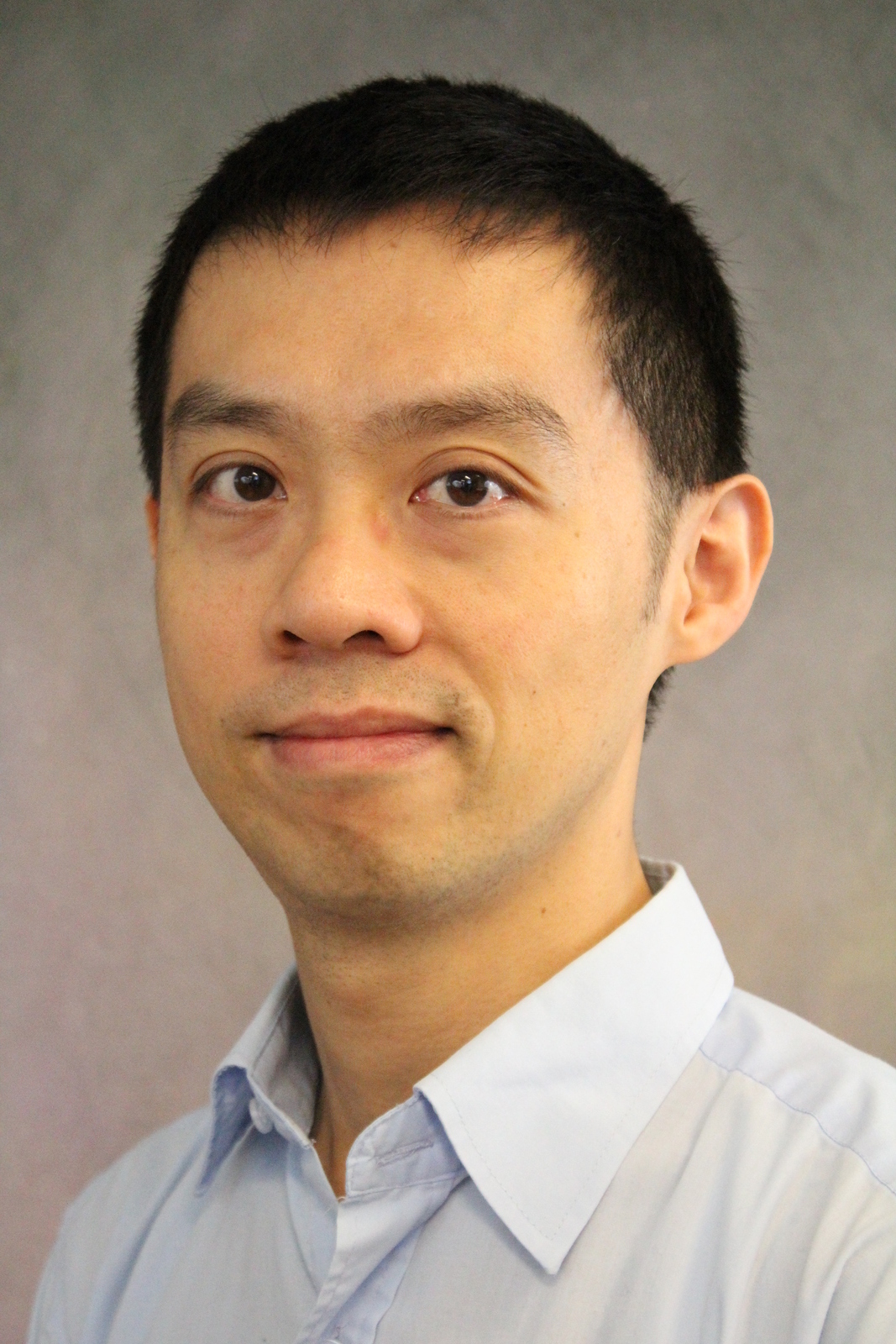
Modular Neural Networks for Low-Power Image Classification on Embedded Devices
Yung-Hsiang Lu, Purdue University link
Embedded devices are generally small, battery-powered computers with limited hardware resources. It is difficult to run Deep Neural Networks (DNNs) on these devices, because DNNs perform millions of operations, and consume significant amounts of energy. Prior research has shown that a considerable number of a DNN’s memory accesses and computation is redundant when performing tasks like image classification. To reduce this redundancy and thereby reduce the energy consumption of DNNs, we introduce the Modular Neural Network-Tree (MNN-Tree) architecture. Instead of using one large DNN for the classifier, this architecture uses multiple smaller DNNs (called modules) to progressively classify images into groups of categories based on a novel visual similarity metric. Once a group of categories is selected by a module, another module then continues to distinguish among the similar categories within the selected group. This process is repeated over multiple modules until we are left with a single category. The computation needed to distinguish dissimilar groups is avoided, thus reducing redundant operations, memory accesses, and energy. Experimental results using several image datasets reveal the effectiveness of our proposed solution to reduce memory requirements by 50%-99%, inference time by 55%-95%, energy consumption by 52%-94%, and the number of operations by 15%-99% when compared with existing DNN architectures, running on two different embedded systems: Raspberry Pi 3 and Raspberry Pi Zero.
Dr. Yung-Hsiang Lu is a professor at the School of Electrical and Computer Engineering at Purdue University, West Lafayette, Indiana, USA. He is the inaugural director of Purdue’s John Martinson Entrepreneurship Center. He is a Distinguished Scientist of the ACM. He received the PhD. from Stanford University and BS from the National Taiwan University. Computing Reviews said, “If you land on a desert island that has a Linux computer, this is the one book to have with you.” about his book “Intermediate C Programming” (CRC Press).
15:20 - 15:55
Invited Talk
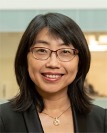
Efficient Deep Learning At Scale
Hai Li, Duke University link
Though the research on hardware acceleration for neural networks has been extensively studied, the progress of hardware development still falls far behind the upscaling of DNN models at the software level. The efficient deployment of DNN models emerges as a major challenge. For example, the massive number of parameters and high computation demand make it challenging to deploy state-of-the-art DNNs onto resource-constrained devices. Compared to inference, training a DNN is much more complicated and has more significant computation and communication intensity. A common practice is distributing the training on multiple nodes or heterogeneous accelerators, while the balance between the data processing and exchange remains critical. We envision that software/hardware co-design for efficient deep learning is necessary. This talk will present our latest explorations on DNN model compression, architecture search, distributed learning, and corresponding optimization at the hardware level.
Hai “Helen” Li is Clare Boothe Luce Professor and Associate Chair for Operations of the Department of Electrical and Computer Engineering at Duke University. She received her B.S and M.S. from Tsinghua University and Ph.D. from Purdue University. At Duke, she co-directs Duke University Center for Computational Evolutionary Intelligence and NSF IUCRC for Alternative Sustainable and Intelligent Computing (ASIC). Her research interests include machine learning acceleration and security, neuromorphic circuit and system for brain-inspired computing, conventional and emerging memory design and architecture, and software and hardware co-design. She received the NSF CAREER Award, the DARPA Young Faculty Award, TUM-IAS Hans Fischer Fellowship from Germany, ELATE Fellowship, eight best paper awards and another nine best paper nominations. Dr. Li is a fellow of IEEE and a distinguished member of ACM. For more information, please see her webpage at http://cei.pratt.duke.edu/.
15:55 - 16:00
Break
Break
16:00 - 16:35
Invited Talk
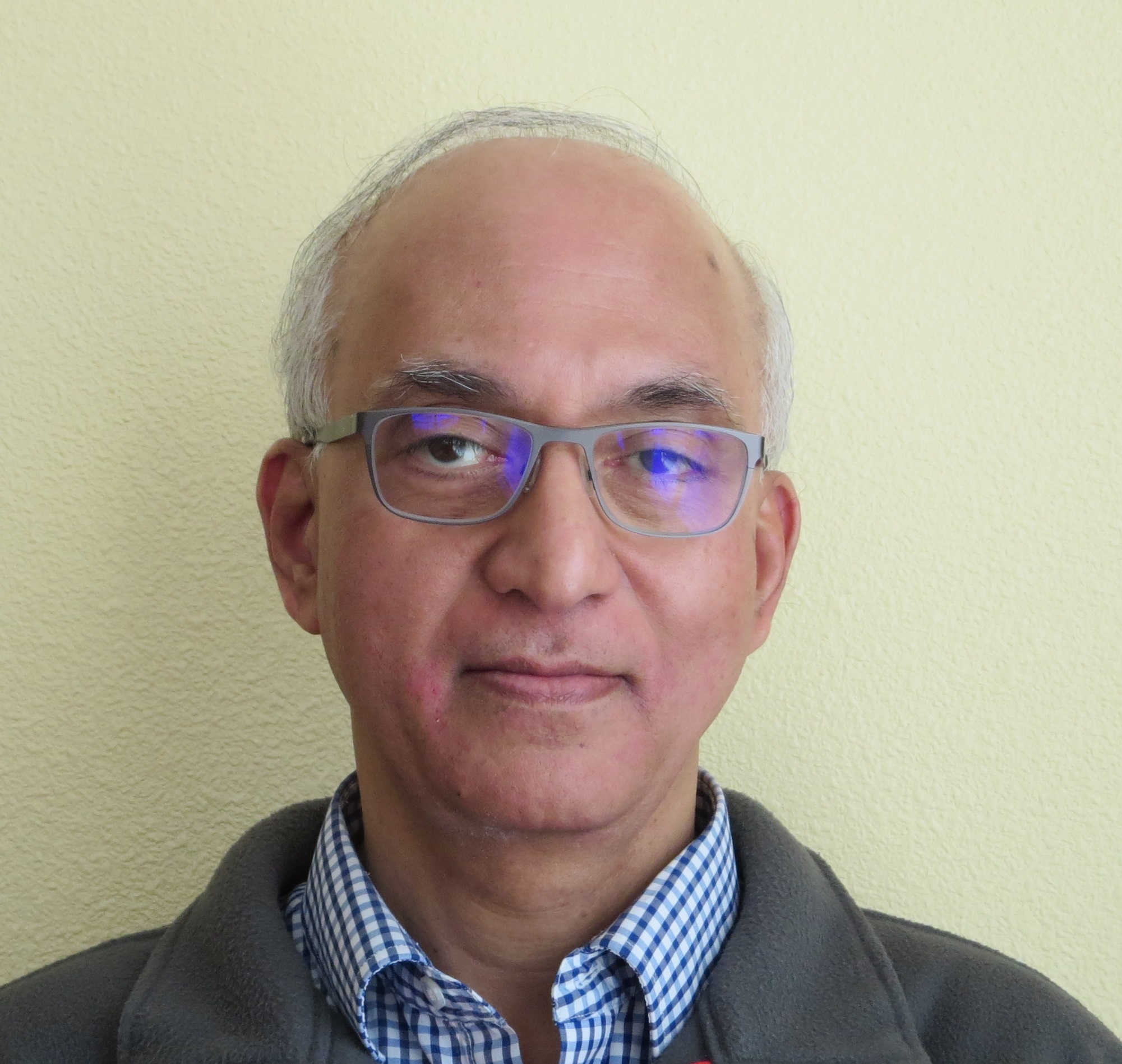
Convergence of Artificial Intelligence, High Performance Computing, and Data Analytics on HPC Supercomputers
Vikram Saletore, Intel Corporation link
Driven by an exponential increase in the volume and diversity of data during the past 15 years, we observe that Data Analytics (DA) and High Performance Computing (HPC) workloads share the same infrastructure. The same convergence is also witnessed with Artificial Intelligence (AI) and HPC and with DA and AI workloads due to the rapid development and use of deep learning frameworks in modeling and simulation algorithms. This convergence has begun to reshape the landscape of scientific computing and enabling scientists address large problems in ways that were not possible before. We present the three pillars that are driving the convergence of AI, HPC, and DA. We will present how the software stacks are supported efficiently over a versatile CPU datacenter infrastructure. We will present AI use cases on large Intel Xeon HPC infrastructure in collaborations with SURF, CERN Open Labs, and Novartis. The use cases include training AI models in histopathology, astrophysics, predicting molecules in chemical reactions, high content screening of phenotypes, and replacing and accelerating HPC simulations in High Energy Physics. We will show scaling of AI workloads significantly reducing the time to train and improving inference performance using Intel DL Boost for quantization.
Dr. Vikram Saletore is a Principal Engineer, Sr. IEEE Member, and AI Performance Architect focused on Deep Learning (DL) performance. He collaborates with industry Enterprise/Government, HPC, & OEM customers on DL Training and Inference. Vikram is also a Co-PI for DL research and customer use cases with; SURF, CERN, Taboola, Novartis, & GENCI. Vikram has 25+ years of experience and has delivered optimized software to Oracle, Informix, and completed technical readiness for Intel’s 3D-XPoint memory via performance modeling. As a Research Scientist with Intel Labs, he led collaboration with HP Labs, Palo Alto for network acceleration. Prior to Intel, as a tenure-track faculty in Computer Science at Oregon State University, Corvallis, Oregon, Vikram led NSF funded research in parallel programming and distributed computing directly supervising 8 students (PhD, MS). He also developed CPU and network products at DEC and AMD. Vikram received his MS from Berkeley & PhD in EE in Parallel Computing from University of Illinois at Urbana-Champaign. He holds multiple patents issued, 3 patents in AI pending, ~60 research papers and ~40 white papers, blogs specifically in AI, Machine Learning Analytics, and Deep Learning.
16:35 - 17:10
Invited Talk
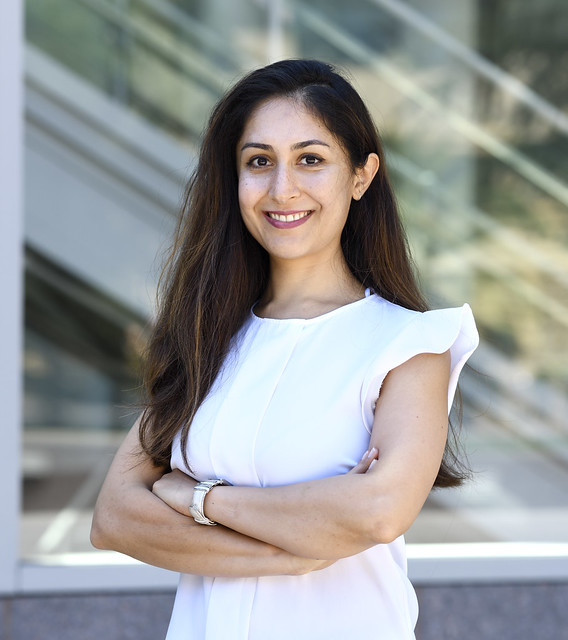
Efficient Machine Learning via Data Summarization
Baharan Mirzasoleiman, Computer Science, University of California, Los Angeles link
Large datasets have been crucial to the success of modern machine learning models. However, training on massive data has two major limitations. First, it is contingent on exceptionally large and expensive computational resources, and incurs a substantial cost due to the significant energy consumption. Second, in many real-world applications such as medical diagnosis, self-driving cars, and fraud detection, big data contains highly imbalanced classes and noisy labels. In such cases, training on the entire data does not result in a high-quality model.
In this talk, I will argue that we can address the above limitations by developing techniques that can identify and extract the representative subsets from massive datasets. Training on representative subsets not only reduces the substantial costs of learning from big data, but also improves their accuracy and robustness against noisy labels. I will present two key aspects to achieve this goal: (1) extracting the representative data points by summarizing massive datasets; and (2) developing efficient optimization methods to learn from the extracted summaries. I will discuss how we can develop theoretically rigorous techniques that provide strong guarantees for the quality of the extracted summaries, and the learned models’ quality and robustness against noisy labels. I will also show the applications of these techniques to several problems, including summarizing massive image collections, online video summarization, and speeding up training machine learning models.
Baharan Mirzasoleiman is Assistant Professor in Computer Science Department at UCLA. Her research focuses on developing new methods that enable efficient machine learning from massive datasets. More specifically, I am interested in designing techniques that can gain insights from the underlying data structure by utilizing complex and higher-order interactions between data points. The extracted information can be used to efficiently explore and robustly learn from datasets that are too large to be dealt with by traditional approaches. My methods have immediate application to high-impact problems where massive data volumes prohibit efficient learning and inference, such as huge image collections, recommender systems, Web and social services, video and other large data streams. Before joining UCLA, she was a postdoctoral research fellow in Computer Science at Stanford University working with Jure Leskovec. I received my Ph.D. in Computer Science from ETH Zurich advised by Andreas Krause. I received an ETH medal for Outstanding Doctoral Thesis, and was selected as a Rising Star in EECS by MIT.
17:10 - 17:55
Panel
Towards Million-fold Efficiency in AI
Training the largest known transformer models consume megawatts of power. Yet, we are far less capable compared to the human brain. In this panel, we will ask: What will it take to train models that understand natural languages like our brain can. Would we continue to increase the energy footprint or innovate and find efficient solutions that consume far less and can reach the illustrious capabilities of the human brain?
Moderator: Satyam Srivastava