Review Committee
- Raj Parihar, Meta
- Satyam Srivastava, D-Matrix
- Sushant Kondguli, Meta
- Ananya Pareek, Google
- Eric Qin, Meta
- Gaurav Jain, D-Matrix
- Abhishek Singh, Micron Technology
Organizing Committee
Raj Parihar link
Meta
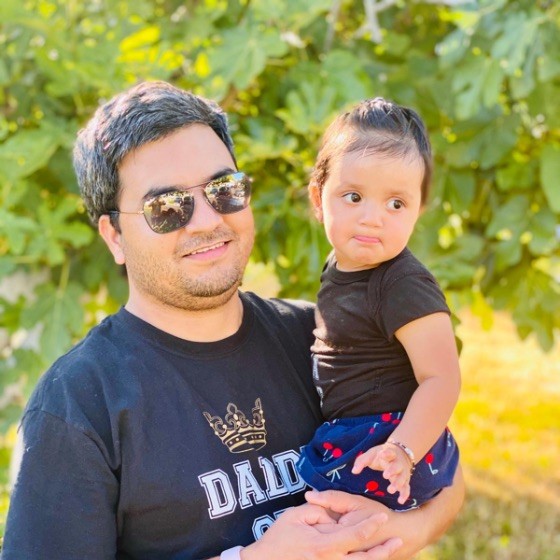
Dr. Raj Parihar is a Technical Lead Manager at Meta in the Infra Silicon ASIC group. Previously, he was the Chief AI Architect at d-Matrix and help defined the flagship product Corsair. Prior to that, Dr. Parihar was part of Microsoft Silicon Engineering and Solutions (SES) group and worked on future generation of Brainwave systems and at Cadence/Tensilica, he was involved in architectural exploration and performance analysis of neural network AI processor DNA 100. He also contributed to the microarchitectural enhancements (next generation branch predictors and cache prefetchers) of P-series Warrior cores at MIPS/ImgTech. His work on Cache rationing won the best paper award at ISMM’16. Dr. Parihar received his Doctorate and Masters from University of Rochester, NY and his Bachelors from Birla Institute of Technology & Science, Pilani, India.
Satyam Srivastava link
d-Matrix Corp.
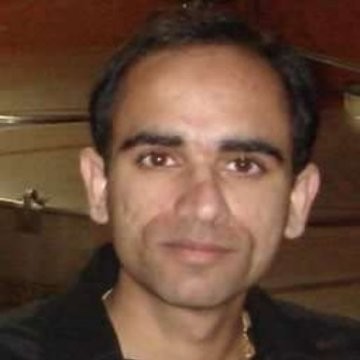
Dr. Satyam Srivastava is the Chief AI Software Architect at d-Matrix Corporation. He works on building the software stack for new AI accelerators. In his prior role at Intel he worked on enabling machine learning and media systems on Intel compute architectures. His interests include machine learning, visual computing, and compute accelerators. Dr. Srivastava obtained his Doctorate degree from Purdue university (West Lafayette, IN) and Bachelor’s degree from Birla Institute of Technology and Science, Pilani (India).
Ananya Pareek link
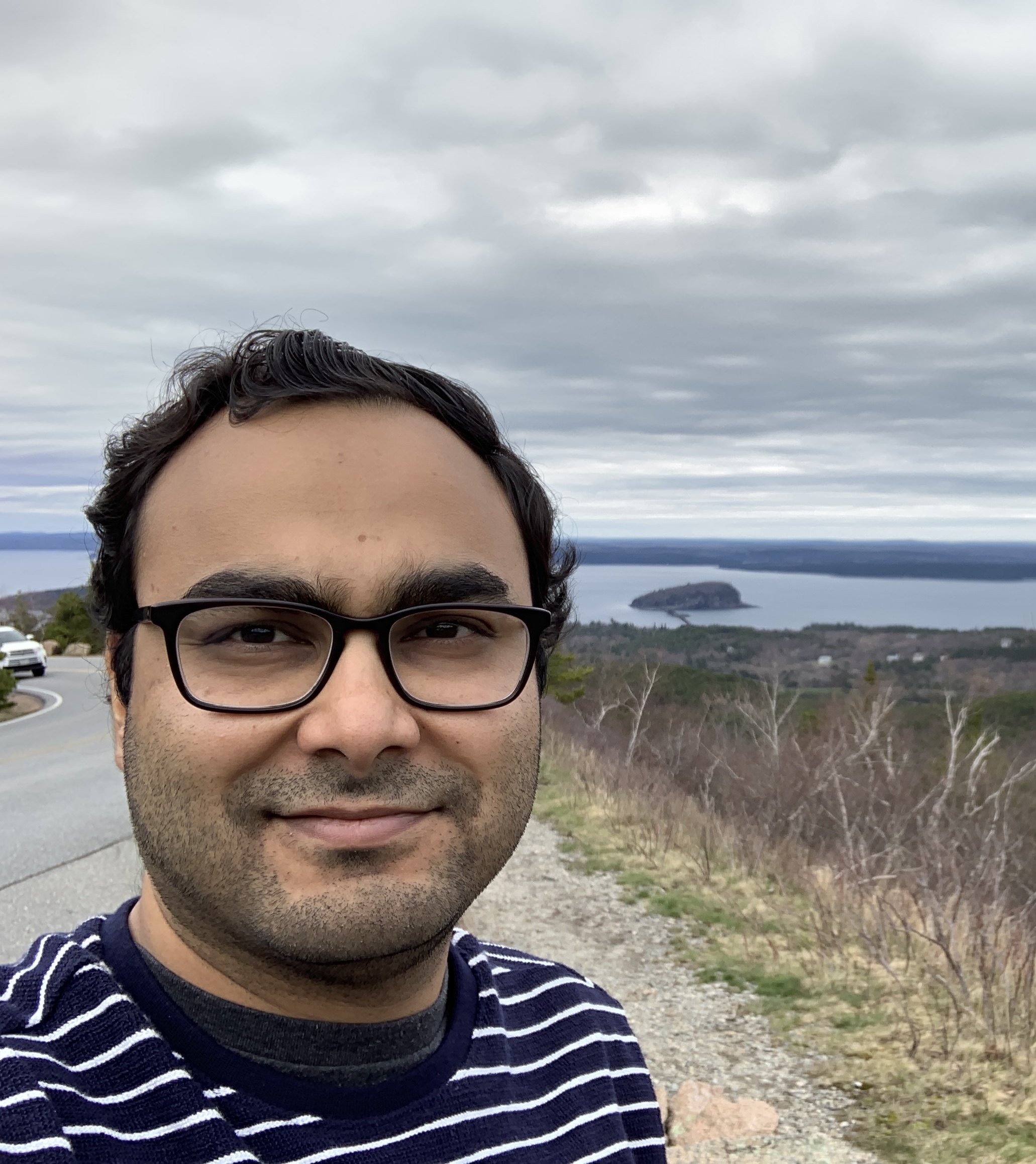
Ananya Pareek is an ML Performance and Co-design Engineer at Google. Currently working on optimizing hardware platforms from a performance-power tradeoff perspective. Previously he has worked at Apple on System Performance Architecture and Modeling and Samsung on GPU Shader and CPU core pipeline and ISA extensions for enabling faster ML/Graphics computations. His interests are in developing hardware platforms for ML/Deep Learning, HW/SW codesign, and modeling systems for optimization. He received his M.S. degree from the University of Rochester, NY, and B.Tech. from Indian Institute of Technology Kanpur, India.
Fanny Nina Paravecino link
Microsoft
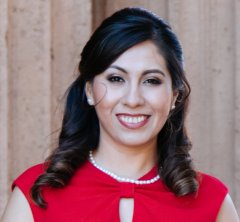
Fanny Nina-Paravecino is currently a Principal Research Eng Manager at Microsoft AI Frameworks, where she leads an interdisciplinary team in Microsoft Azure operating at the intersection of high-performance computing and artificial intelligence (AI) to deliver the fastest AI execution at the cloud scale.
Sushant Kondguli link
Meta
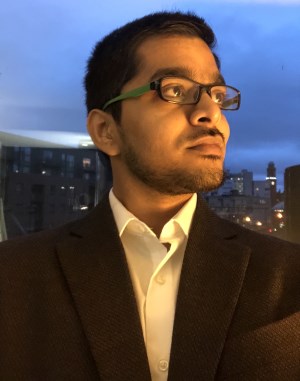
Dr. Sushant Kondguli is a Graphics Architect at Meta Reality Labs Research where his research focuses on low power architectures for on-head rendering devices. Prior to that, Dr. Kondguli was a Mobile GPU architect at the Advanced Computing Lab of Samsung where he helped develop the XClipse GPU architecture used in Samsung’s flagship galaxy smartphones. He received in PhD and B.Tech. degrees from University of Rochester and IIT Kharagpur, respectively.
Eric Qin link
Meta
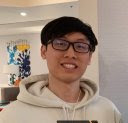
Eric Qin is an ASIC Architecture Engineer at Meta where he works on developing the next generation architectures for ML workloads. Prior to that, Eric received his PhD in ECE at Georgia Institute of Technology and Bachelors from Arizona State University. His research interests include computer architecture and domain specific accelerators.
Gaurav Jain link
d-Matrix
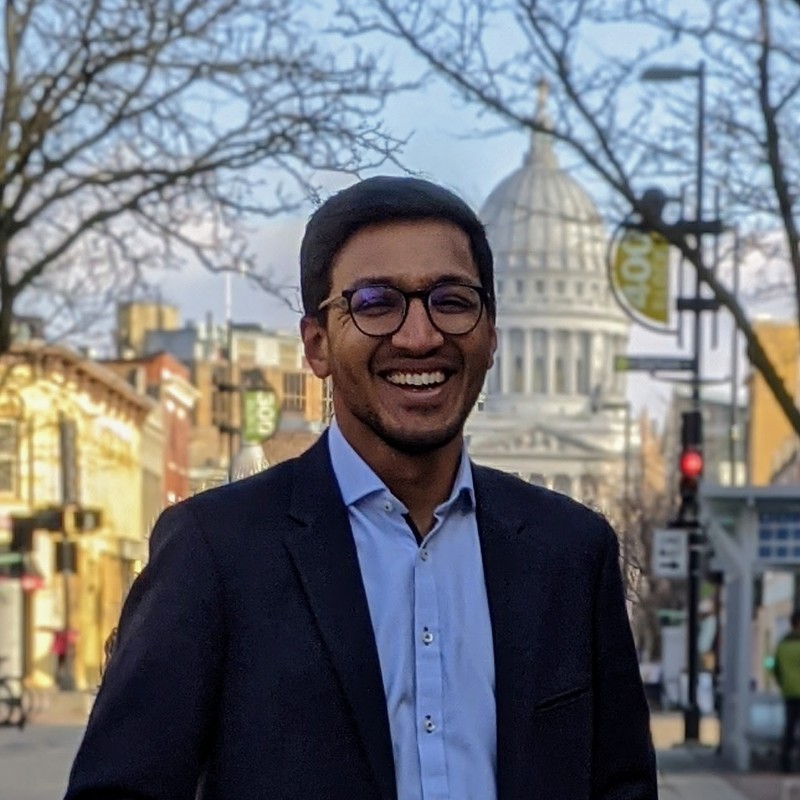
Gaurav Jain is a software engineer at d-Matrix where he is leading the efforts for building the kernel software stack for next-generation in-memory compute LLM inference hardware. Along with this, he’s also involved in the research and exploration of techniques aiming towards the improvement of end-to-end model performance and reducing memory overhead. Prior to this he was a silicon architect in the Google Pixel TPU machine learning accelerator team where his day-to-day activities involved architecture specification, performance modeling, and workload characterization for Google’s machine learning workloads. Gaurav holds a Master’s in Electrical and Computer Engineering from the University of Wisconsin-Madison and his research interests span across multiple domains including model optimization, ML Systems, hardware-software codesign, and computer architecture.